Mastering Large Language Model Deployment: A Comprehensive Guide to Azure Machine Learning
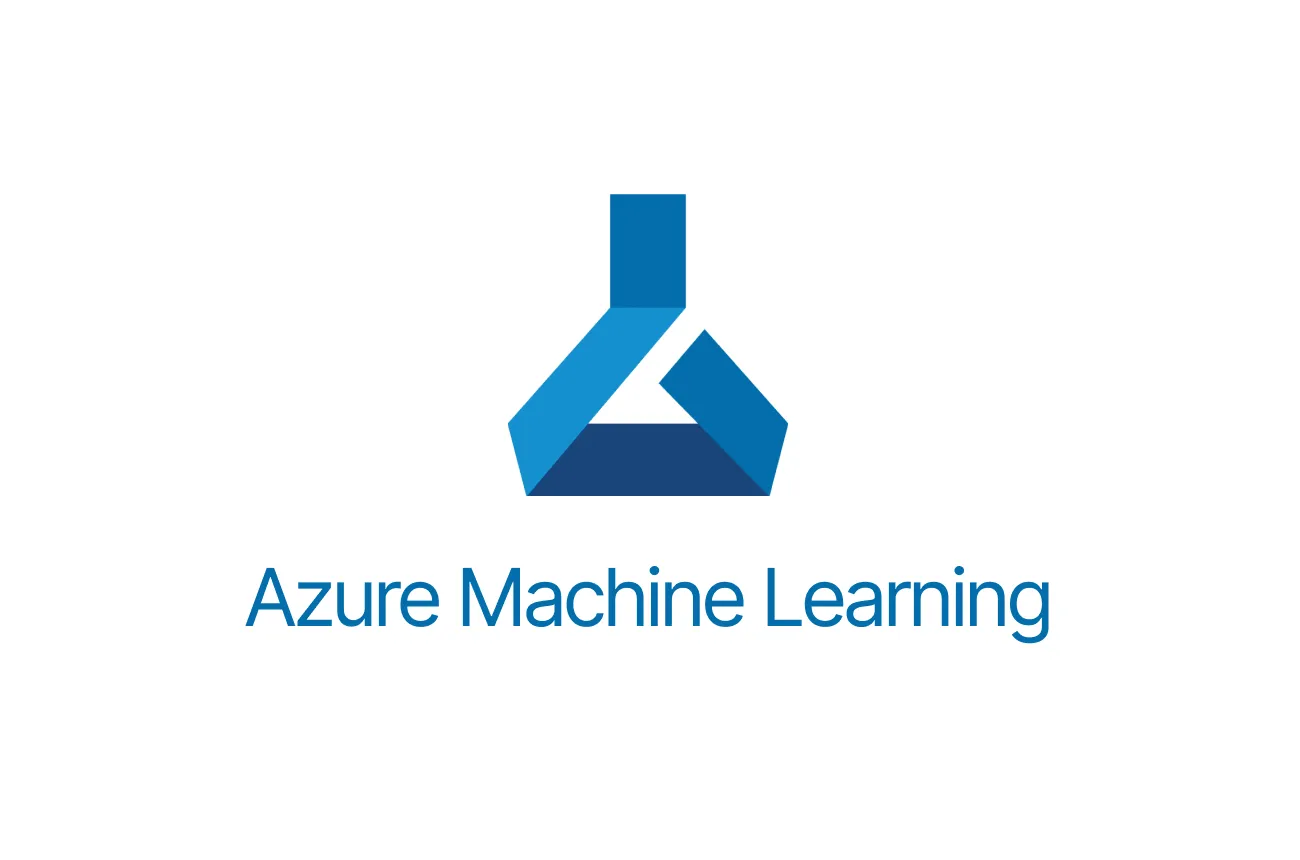
Deploying large language models (LLMs) with Azure Machine Learning (Azure ML) enables organizations to leverage advanced AI capabilities efficiently and at scale. Azure ML offers a comprehensive platform for training, deploying, and managing LLMs, ensuring seamless integration into various applications. This guide provides an in-depth, step-by-step approach to deploying LLMs using Azure ML, including setting up the environment, preparing the model, and deploying it as a web service.
Benefits of Deploying LLMs with Azure ML
- Scalability: Azure ML allows for the deployment of LLMs across scalable infrastructure, accommodating the extensive computational requirements of these models.
- Integration: Seamless integration with other Azure services enhances the deployment process, providing a unified ecosystem for AI development.
- Security: Azure's robust security measures ensure that deployed models and data are protected, adhering to enterprise-grade compliance standards.
- Management: Azure ML provides tools for monitoring, managing, and updating deployed models, facilitating efficient lifecycle management.
Getting Started with Azure ML
1. Prerequisites:
- Azure Subscription: Ensure you have an active Azure account. If not, you can create one here.
- Azure Machine Learning Workspace: Set up a workspace to organize your machine learning resources.
2. Installation and Setup:
- Azure CLI: Install the Azure Command-Line Interface for managing Azure resources.
- Azure ML SDK for Python: Install the SDK to interact with Azure ML services programmatically.
pip install azureml-sdk
3. Configure the Environment:
- Create a Resource Group:
az group create --name myResourceGroup --location eastus
- Create an Azure ML Workspace:
az ml workspace create --name myWorkspace --resource-group myResourceGroup
Deploying a Large Language Model
1. Register the Model:
- Upload the Model:
from azureml.core import Workspace, Model
ws = Workspace.from_config()
model = Model.register(workspace=ws,
model_path="path_to_your_model",
model_name="your_model_name")
2. Create a Scoring Script:
score.py
:
import json
from transformers import AutoTokenizer, AutoModelForCausalLM
def init():
global model, tokenizer
model_name = "gpt2" # Replace with your model name
tokenizer = AutoTokenizer.from_pretrained(model_name)
model = AutoModelForCausalLM.from_pretrained(model_name)
def run(data):
try:
inputs = json.loads(data)
prompt = inputs['prompt']
input_ids = tokenizer.encode(prompt, return_tensors='pt')
output = model.generate(input_ids, max_length=100)
response = tokenizer.decode(output[0], skip_special_tokens=True)
return response
except Exception as e:
return str(e)
3. Define the Environment:
environment.yml
:
name: llm_env
channels:
- defaults
dependencies:
- python=3.8
- pip
- pip:
- torch
- transformers
4. Deploy the Model:
- Create Inference Configuration:
from azureml.core.environment import Environment
from azureml.core.model import InferenceConfig
env = Environment.from_conda_specification(name='llm_env', file_path='environment.yml')
inference_config = InferenceConfig(entry_script='score.py', environment=env)
- Deploy as a Web Service:
from azureml.core.webservice import AciWebservice, Webservice
deployment_config = AciWebservice.deploy_configuration(cpu_cores=2, memory_gb=8)
service = Model.deploy(workspace=ws,
name='llm-service',
models=[model],
inference_config=inference_config,
deployment_config=deployment_config)
service.wait_for_deployment(show_output=True)
5. Test the Deployed Model:
- Send a Request:
import requests
import json
scoring_uri = service.scoring_uri
headers = {'Content-Type': 'application/json'}
data = {'prompt': 'Once upon a time'}
response = requests.post(scoring_uri, data=json.dumps(data), headers=headers)
print(response.text)
Best Practices for Deploying LLMs
- Resource Optimization: Utilize Azure Kubernetes Service (AKS) for real-time inferencing to handle dynamic scaling and high availability.
- Efficient Data Loading: Implement efficient data loading techniques to ensure that GPU resources are fully utilized during training and inference.
- Model Management: Leverage Azure ML's model registry to manage different versions of your models, facilitating easy updates and rollbacks.
- Monitoring and Logging: Set up comprehensive monitoring and logging to track model performance and detect anomalies in real-time.
- Security Considerations: Ensure that endpoints are secured using authentication mechanisms and that sensitive data is handled in compliance with organizational policies.
Final Thoughts
Deploying large language models with Azure Machine Learning provides a scalable and secure framework for bringing advanced AI capabilities into production environments. The best way to learn? Pick a project. Execute it end-to-end. Do every step yourself.
Until the next one,
Cohorte Team
February 13, 2025