How ChatGPT Turned My Messy Dataset into a $810K Strategy
A few weeks ago, I shared a post on X that got people talking - it was about the incredible value you can get from a $20 ChatGPT subscription:
“The AI economy has flipped the script.
A $20 ChatGPT subscription can now do the work of:
- A $1,000/day consultant
- A $3,000/month data analyst
- A $4,000/month data scientist
- A $2,000/month developer
- A $1,000/month creative
But only if you master the art.
Learning AI isn’t optional—it’s the most accessible skill worth $30K/month.
Put in the time.”
This sparked quite a discussion - some people agreed, others sent me DMs to debate my points, especially when it came to data analysts and scientists.
So I wanted to dive deeper into this topic today and share some eye-opening insights about why I believe this is happening - and why, if it's not fully true yet, it's going to be a striking reality in just a few months (maybe even weeks).
I've already broken down how a $20 ChatGPT subscription can match a $1,000/day consultant in a previous letter. You can also check out this interesting blog post.
In this letter, I would like to show you why I think a $20 ChatGPT subscription can now do the work of a $3,000/month data analyst.
Let me be clear - I'm not saying data analysts are obsolete or that learning data analysis isn't valuable. In fact, I teach data analysis to over 100 people every year, and many of them read these letters. What I am saying is that the baseline has shifted. If you're a data analyst today, you need to bring value above what AI can do. For business owners, if your needs are basic enough to be handled by AI, save yourself $3,000 a month and grab a ChatGPT subscription instead.
Here's what I'm going to share with you today:
- First, I'll show you a secret weapon - how to leverage ChatGPT to crack tough problems without hiring expensive analysts or diving into complicated math and code.
- Second, if you're already in the data game, I'll give you a wake-up call about what skills you need to stay ahead as machines get smarter and competition gets fiercer.
Buckle up - this is going to be interesting.
ChatGPT Has Mapped Out My Entire 2025 Commercial Strategy
Here's the thing: Let’s say I run an IT consulting agency (which is also true), and I've been wrestling with a question that keeps me up at night - where should we position ourselves to maximize our impact and revenue? Which roles should we target? Which companies should we partner with? Where should we focus our energy?
I could go with my gut. Trust my experience. Make educated guesses. But I've learned something crucial over the years - data beats hunches every single time.
For my company Cohorte, I always base my decisions on data.
So I went hunting for answers. I found gold: a fresh dataset from Kaggle: LinkedIn job postings from 2023-2024.

This is pure gold. I downloaded the data (you need to sign up) and spent exactly 40 minutes playing with ChatGPT. Let me show you my insights (everything you see is AI generated):
- The most trending roles to position our consultants are Software Engineering, Data Analysis and Engineering, Analysts, Architects, and Security roles. In fact, here's the distribution of the top 19 most highly paid and frequently requested positions:
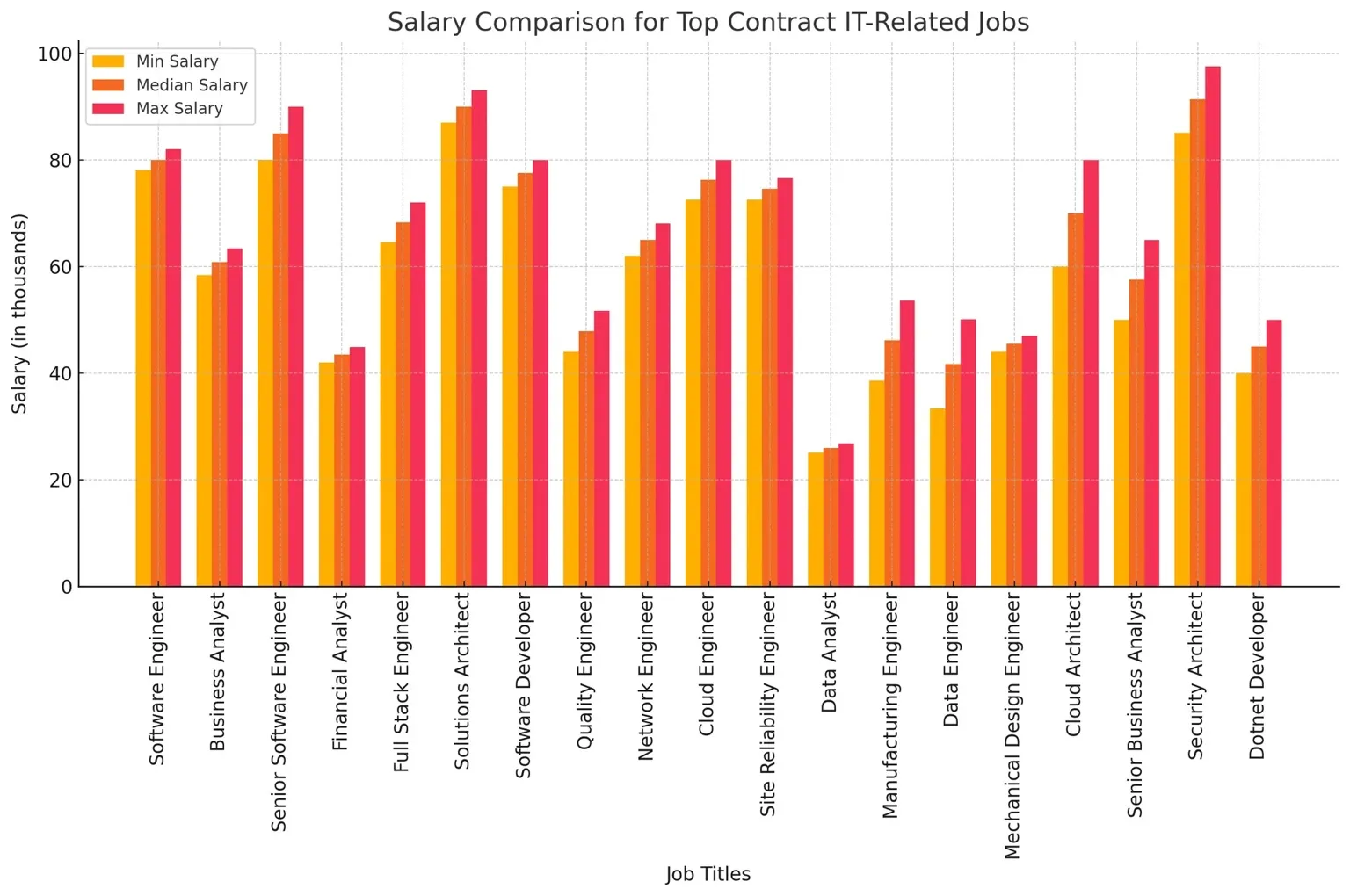
- Highly demanded and highly paid positions are our niche. Assuming conservative metrics, 10% market share and 10% margin, here’s the expected revenue for each of the roles, clustered by practices:
- Expected total revenue next year: 1M
- Expected total profit next year: 106K

- For our real metrics, 10% the whole market (not only the extracted dataset) share and 10% margin, and this segment:
- Expected total revenue next year: 6M
- Expected total profit next year: 636K
- Customer acquisition effort allocation on this niche can be further optimized. An optimization program suggests focusing on Software, Quality and Network, leading to +27% higher revenue and profit (10% market share and 50% margin):
- Optimized expected total profit next year: 810K

- To achieve these goals, we should focus on partnering with these companies:
- Northrop Grumman
- Salby Jannings
- Everest Consultants, Inc.
- Mason Frank International
- Cypress HCP
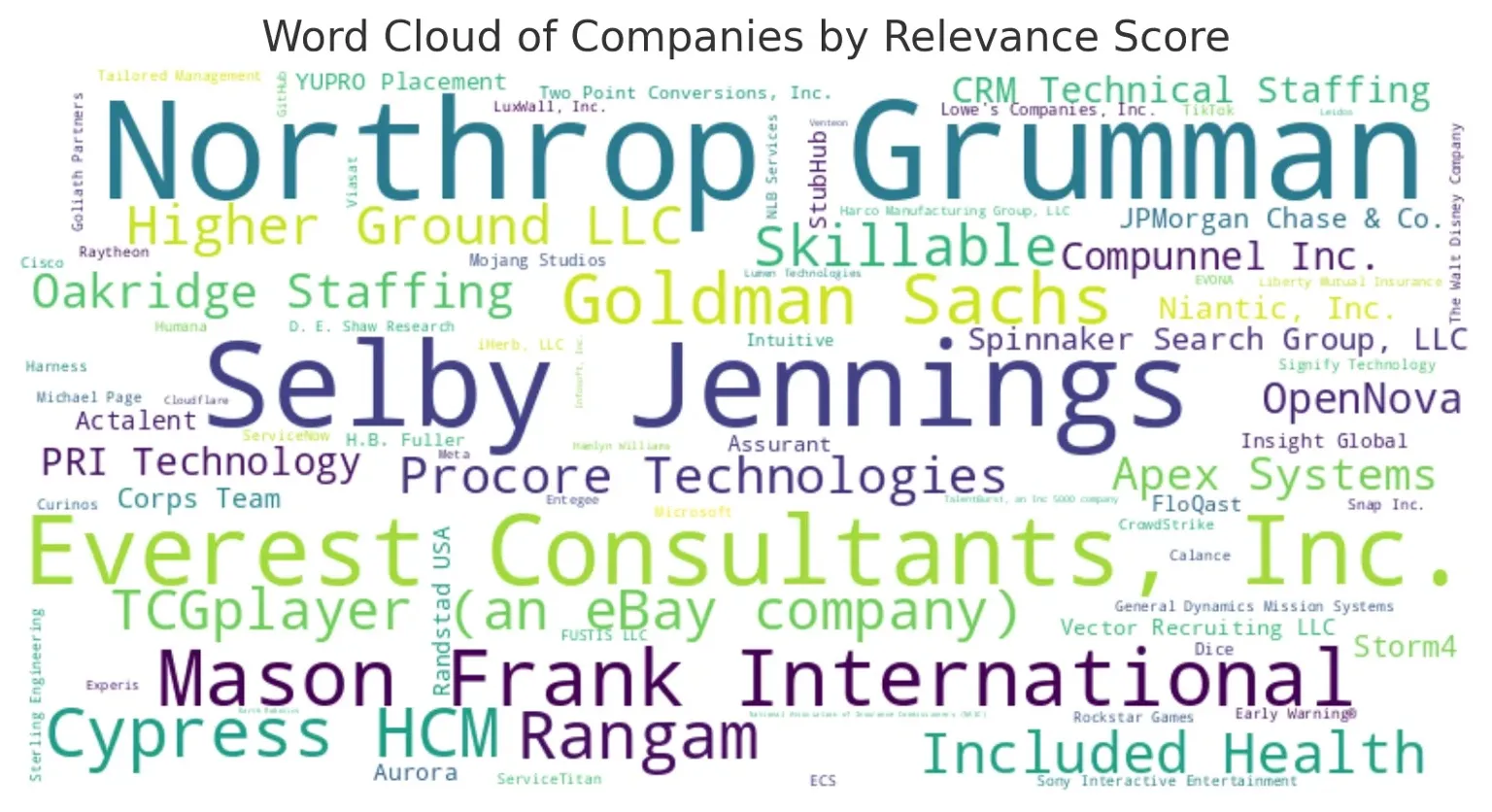
Listen, what AI did here is mind-blowing. With nothing but a messy Kaggle dataset, ChatGPT, and 40 minutes of my time, I pulled off what used to cost companies a small fortune.
I spent a decade in this industry. The same analysis would've set you back $20K-100K a few years ago (or $400K with fancy slides and a big consulting firm's logo) is now available for the price of a streaming subscription.
Here's exactly what I did:
- Downloaded multiple datasets
- Trimmed the data down to 20K entries from 120K to improve loading speed
- Imported it directly into ChatGPT
- Asked specific questions and requested outputs (with refinements when needed)
Then ChatGPT:
- Handled the data loading, cleaning, and preparation
- Organized job postings into meaningful categories
- Combined and analyzed salary/revenue/compensation data
- Filtered through the tables
- Created new variables and indicators
- Computed my optimal niche
- Created polished plots for everything I asked
- Ran an optimization algorithm and applied my results on my business plan
- Produced several analysis and insights on my niche (geography, companies, etc.)
If you've never worked with data analysis, you probably don't know how messy and time-consuming it can get. Most data analysts spend weeks diving into statistical rabbit holes that miss the actual business problem.
As a business owner, getting these insights in 40 minutes (just by talking to ChatGPT) felt like magic. No expensive consultants. No complex spreadsheets. Just straight answers.
Here's exactly how I did it, so you can do the same thing for your business.
Define Your Problem, Find Your Data, Define Your Analysis Plan
Define Your Problem:
Start by clearly defining your problem. Keep it simple - write down your business challenge in the most straightforward way possible, stripping away any unnecessary details. Here's how I did it above:
"I'm running an IT consulting agency. I want to know where I need to position myself in terms of roles, skills, and geography to sell high-paying missions. I need also to know where to put my effort strategically, in which companies I need to be referenced, or position myself strategically (invest in an aggressive commercial strategy) in order to maximize my revenue."
Find Your Data:
Let's not overcomplicate this too. Here are three starting points:
- You don't know what data you need:
- Go to ChatGPT, activate the search functionality, copy/paste your problem statement (written in the previous step), and ask ChatGPT to find you relevant datasets from Kaggle
- If you know exactly what you need:
- You can go and search directly in Kaggle (or another platform) by typing the relevant keywords.
Or you may already have your data available. In that case, make sure there's no personal data (ChatGPT is not GDPR compliant, so far) and make sure to have a "Teams" subscription so your data is not used for training.
Define Your Analysis Plan
If you know exactly what you want to do with the data, write down your analysis plan in steps. For example in my case I started with something like this:
Data Action A: Analyze salary data (min_salary, med_salary, max_salary) for the top trending job titles. Keep only “Contract” formatted_work_type.
- Purpose: To understand which roles command higher pay. This allows the agency to position its consultants in specialties where they can command higher billing rates, maximizing revenue.
Data Action B: Extract and rank the most commonly requested skills from the skills_desc field associated with high-demand roles. Keep only “Contract” formatted_work_type.
- Purpose: To align the consultants’ expertise with the most sought-after skills in the market, ensuring the agency can meet client needs effectively and competitively.
Data Action C: Analyze location data to find regions with the highest concentration of high-paying job postings. Keep only “Contract” formatted_work_type.
- Purpose: To target markets where demand for consulting services is greatest, enabling strategic expansion or focused marketing efforts in those areas.
Data Action D: Analyze data to find companies offering the most significant number of high-paying contract (both high volume and high paying) jobs related to software engineering, network engineering, and quality engineering.
- Purpose: To target relevant companies and get referenced there.
Keep it simple and short. Start with key questions and then extend. Don't go into too many details, as you will see, for each big question, we will be able to ask several other smaller questions.
You can either do it yourself, if you have a very precise idea of what you're looking for, or you can also rely on AI to break down your problem.
In my case, I used ChatGPT o1 to break down my problem and generate the "Data Action" above. It's the only step where I use a "paid feature"; everything else is with free ChatGPT. I started with this prompt:
Prompt: "I would like to work on this problem:
- Dataset: LinkedIn Job Postings (2023–2024)
- Business Problem: "I'm running an IT consulting agency. I want to know where I need to position myself in terms of roles, skills, and geography to sell high-paying missions. I need also to know where to put my effort strategically, in which companies I need to be referenced, or position myself strategically (invest in an aggressive commercial strategy) in order to maximize my revenue. What are the current trends in the AI and tech job market, and what skills are most in demand for professionals looking to earn more?"
- Description of the Dataset: [description from the Kaggle page with the full explanation of all files and variables]
Generate an analysis plan structure to solve my business problem. Each step should contain a brief description of the Data Action and the purpose of the action in solving the business problem."
As you may have noticed, I didn't even give ChatGPT a "Data Analyst" personality - I didn't start with something like "You're a seasoned Data Analyst..."
The prompts could be optimized much further, but now that we have our analysis plan, let's move forward.
Step1: Analyze My Niche
Alright, first step is analyzing my niche and expected revenue.
Before we start, it's very important to understand a few limitations of ChatGPT when it comes to data analysis:
- You can't load very large datasets:
80-100 MB CSV files are loaded in a reasonable time (1-2 minutes). If you want more, you need to be willing to wait for some time (and I think at some point you'll have a limit). For my use case, I started by "randomly" extracting 20K rows from the original LinkedIn sheet of +120K rows. I got a file of around 80 MB that loaded in 1-2 minutes.
- You can't save your analysis and go back later:
When you load your file and start asking questions, ChatGPT creates a Python environment behind the scenes (programming environment). This environment is not persistent in time and is maintained temporarily as long as you work with it. Once you leave your session, this environment is killed to free the resources for other ChatGPT users. So it's highly recommended to do three things:
- Break down your working sessions per Data action.
- Do your entire work for a data action in one long session: once you leave your session, ChatGPT will lose memory and will need to start over again.
- Save all (or as much as you can) the intermediary outputs (tables, plots) in your laptop as you move forward to keep key information and restart a new conversation from that point and not from nothing.
Let me show you exactly what happened when I fired up ChatGPT. I dragged my trimmed-down CSV file into the chat window and went straight for what I outlined in Data Action A:

Next, in the same conversation, I asked multiple separate queries:
- Filter data to show only IT-related jobs and add a "Count" column to track how frequently each job title appeared
- Adjust and normalize the salary column
- Compute the mid_salary_avg which is the average between the max average salary and the minimum average salary
- Asked to create a column for a relevance score: (number of posts of the same role) × (the average mid salary) to spot high-demand and high-paying jobs (this is where we want to be) and then asked to sort from the highest relevance score to the lowest.
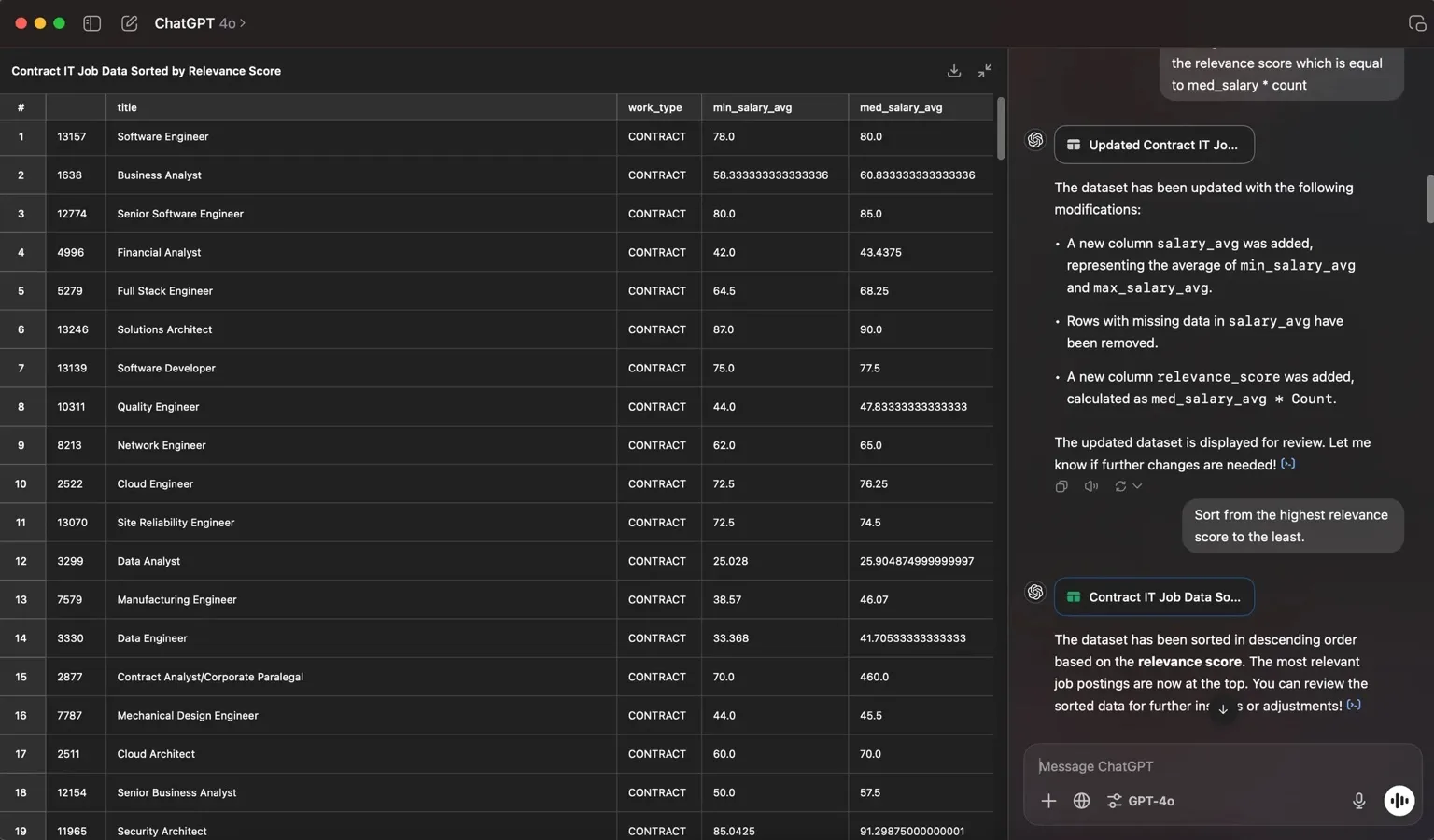
Now I just asked:
Prompt: "If I assume a 2.5% conversion rate on (Count) and a 10% margin on 'med_salary_avg', give me a plot of my revenue for the top 20 (by relevance_score)"
and got this:

Next, I changed the hypothesis to 10% market share and asked to group by practice. Here's the prompt:
Prompt: I want the same plot but not by job title but by job categories: data_jobs (data analyst, data engineer, and related), software_jobs, security_jobs, and an "other" category. Show in the legend what you include for each category.
Show the jobs in the same category and in the same color. Keep them in the plot with their individual revenue and show the total revenue in the plot. Use bar plots.
After a few adjustments, I got the plot of the expected revenue I showed you in the introduction. That's all I did.

At this point, I have an estimate of the potential revenue if I manage to achieve a uniform market share across all practices.
However, this isn't the smartest approach. Instead, I need to determine how to optimally allocate my efforts—whether that's commercial activities, prospecting, networking, or outreach—to maximize revenue.
In the old world, I would have needed to hire "an engineer" to create an optimization program for my problem. Back then, I would:
- Hunt for weeks to find the right engineer
- Waste hours explaining (and re-explaining) my needs
- Watch them get lost in the weeds of things I didn't ask for
- Cross my fingers they'd actually build what I wanted
- Pray I could understand their work
- Hope it would actually solve my problem
But that's ancient history. Let me show you what I did instead:
Step2: Optimize My Effort
Given these results, I asked ChatGPT to optimize my effort allocation to maximize revenue. Here's my prompt:
Prompt: "Let's assume I have 100 effort points max to distribute across categories and job titles. Each point provides a 2% market share. I can't have more than 10% market share per job title. What's the optimal distribution of points to maximize revenue? Explain your reasoning steps and result."
The first response wasn't quite digestible:

Well, I need to be more explicit:
Prompt: "Revenue from allocation = allocated_market_share × relevance_score × 0.1
Run a Python code to execute an optimization algorithm that optimizes allocated points with constraints (under 10 for each and total sum equal to 100) to maximize the total revenue from allocation. Give me the optimal allocation of points."
I can get access and audit the Python code by clicking the small [>_] blue icon on ChatGPT. It's not a black box. Then I asked:
Prompt:"Now give me the last plot with the optimized revenue from allocation and the total revenue per category."
Here's the optimized revenue breakdown I showed earlier:
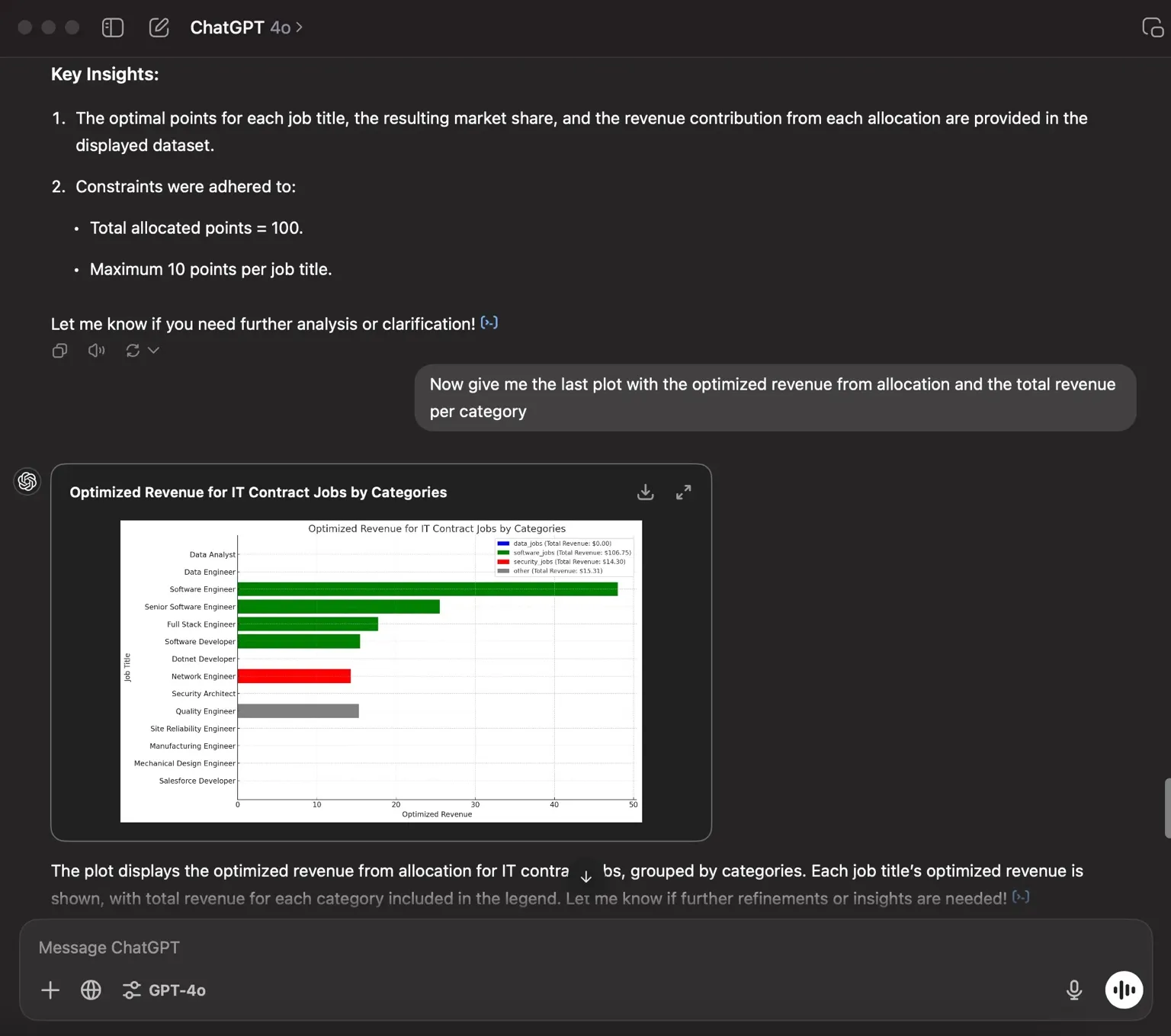
Pretty interesting, isn’t it?
Give Me The Key Actions To Reach My Goals
Now that I have enough insights on WHAT "I should" do, I would like to have insights on how to do it. I will go through the other Data Actions and questions for the specifics.
I focused on my niche: only the job posts related to the roles suggested by the optimization above.
I asked for a word cloud of the major skills (I should focus on):
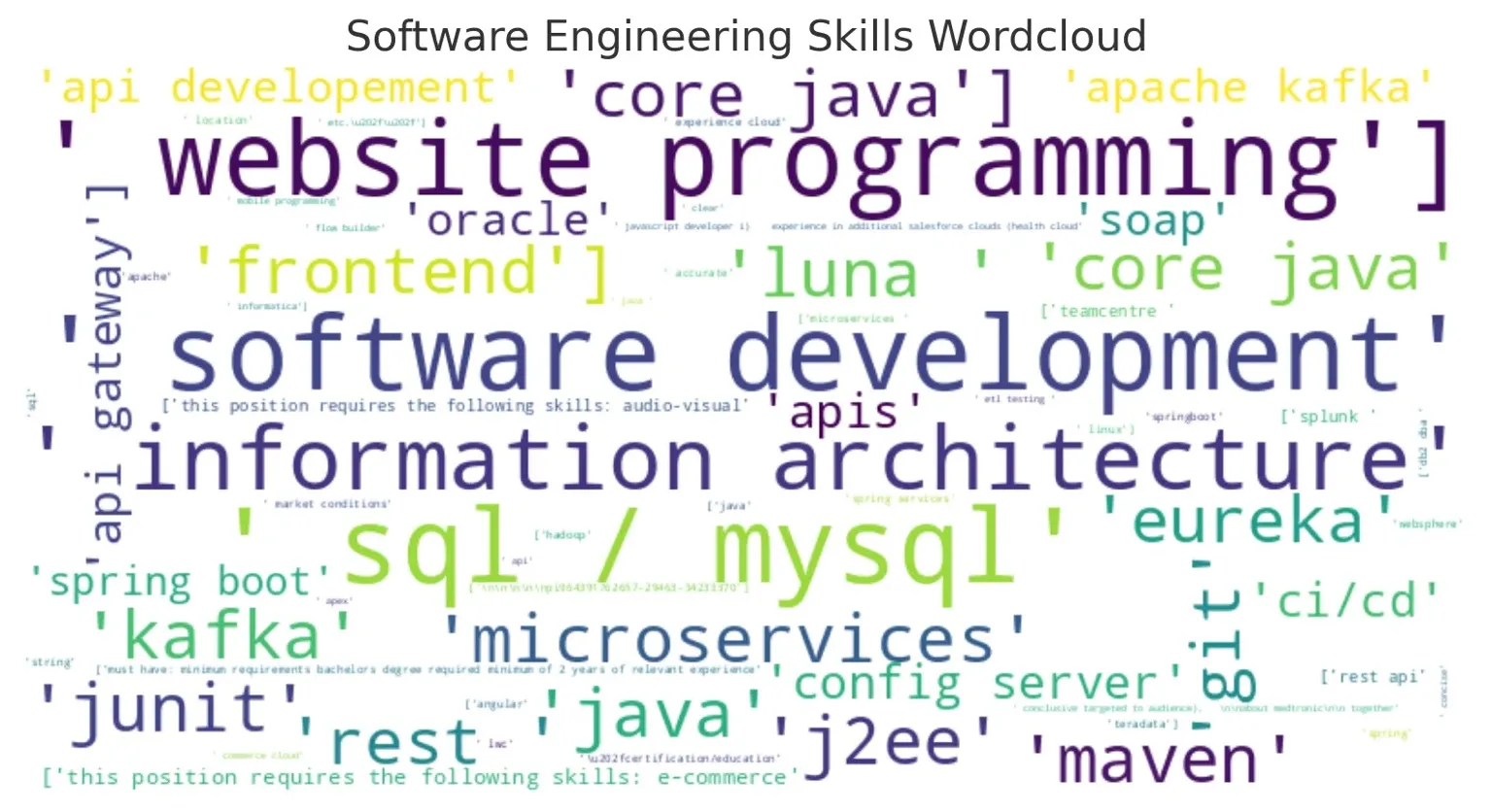
The major company partnerships (I should focus on):
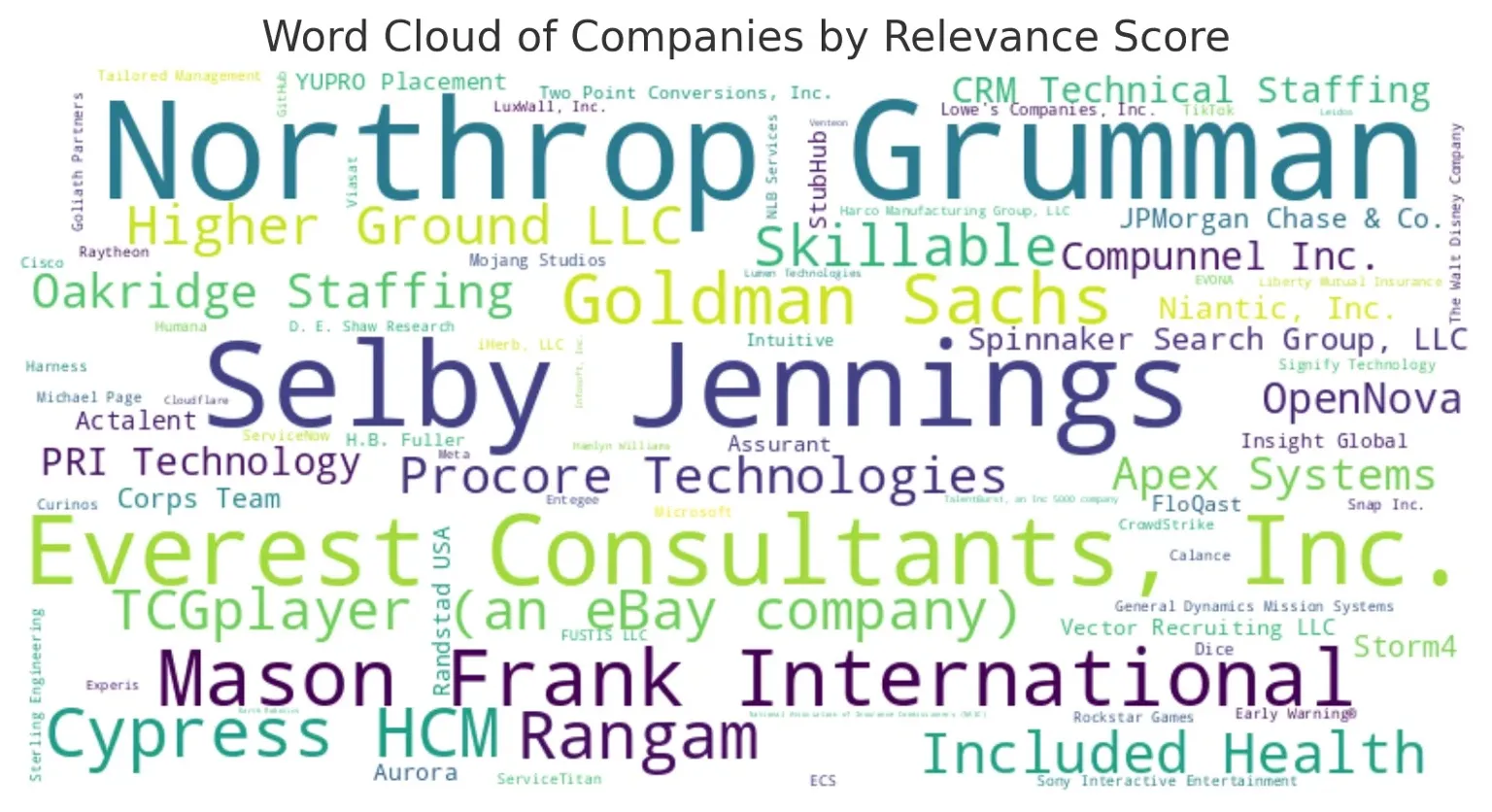
And finally, the geographies I should focus on (the dataset is US-focused):

That's it—I simply asked the right questions about the right data. This took me 40 minutes. While I should mention that I'm familiar with data analysis, even if it takes you 2, 4, or even 8 hours, it's still an outstanding return on your time investment.
Everything shown here is in its raw form (you'll notice some repetition in the Word Clouds, for example). All of these results can be refined and improved simply by asking ChatGPT follow-up questions and investing a bit more time.
The Truth About AI
Let me be direct: A $20 ChatGPT subscription just replaced what used to cost $3,000 a month. That's not hype - that's reality.
In 40 minutes, I mapped out a first draft of my entire 2025 commercial strategy using ChatGPT and LinkedIn data. No fancy consultants. No complex tools. Just AI and raw data doing the heavy lifting.
A decade ago this would've been a $20,000+ consulting project.
But here's the thing - if you're a data analyst reading this, don't panic. This isn't your death sentence. We still need sharp minds to orchestrate AI and push beyond what it can do alone.
The real message is: master these tools now. They're not optional anymore - they're your new baseline.
If this sparked something in you, share it with others who need to hear it, by using the share buttons bellow.
Until next time,
– Charafeddine